The High Costs of Observability: Uncovering the Burden on Modern Systems
Apr 30, 2024
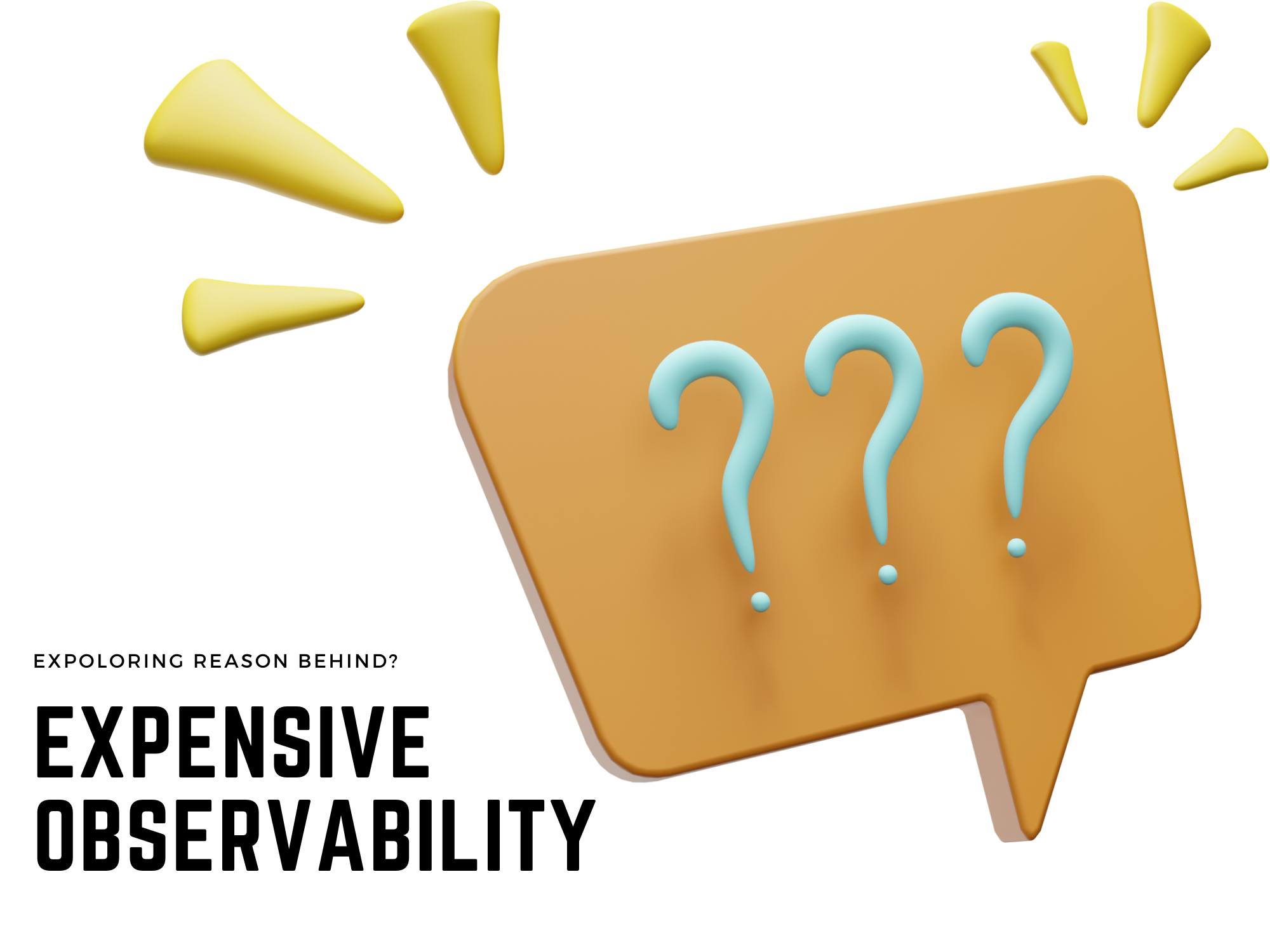
Unveiling the Expense of Observability
Having immersed myself in this domain for more than three years, I’ve continually pondered the enigma of observability’s steep costs. Is it truly necessary for observability tools to incur such significant expenses in both operation and upkeep?
In my quest for answers, I dissected every observability solution into three fundamental components:
- Data Collection
- Data Storage
- Data Analysis
Exploring Data Collection
Data collection stands as the cornerstone of any observability system. In this realm, agents bear the weighty responsibility of tasks ranging from basic up/down monitoring to gathering performance metrics, shipping logs, ensuring file integrity, and fortifying applications with firewalls, among others.
These agents are finely tuned to utilize only a small fraction of computing resources, typically around 0.3% on most platforms. Further optimization would necessitate extensive research and may not yield substantial cost savings.
Understanding Data Storage
Data storage constitutes a significant portion of the cost of an observability tool, presenting two primary challenges:
- Determining what data to store
- Selecting the optimal storage method
Deciding What to Store
Optimal case scenario for this problem would have been a filter that dynamically changes what to store based on how system is performing. For example if system is facing some performance issue it should increase granularity of the data being stored, once system comes back to normal state it should decrease the granularity.
But in most of systems this is not the case and we get a fixed data at all times, so developers have to decide upon what to keep and what not to in order to have balance between storage cost and being able to use this data effectively while solving issue.
Choosing How to Store
Once we have decided on what to store next question that has to be answered becomes how to store it, do we store this in raw format or compressed form, do we store matrices directly or store aggregate values and many more such question. Depending on answers to these questions our storage cost varies significantly.
At CtrlB, we’ve tackled this challenge with finesse, enabling us to offer our solution at a significantly lower cost compared to our competitors. Intrigued? Reach out to us at support@ctrlb.ai, and let us revolutionize your observability costs beyond your wildest expectations!
Extracting Insights from the Observability Data
If we are not able to analyse this data it is useless. Analysing this data is pocket heavy including 2 major expense, reading from storage and then performing operations on this data. Reading cost depends on factor discussed in previous section so let's discuss about cost of processing this data.
Although we don’t have numbers about closed source software, I analysed system requirements of opensource projects such as jaeger, zipkins etc it revolves around 4–8 core CPUs & 8–16GB memory. On putting these parameters into AWS pricing calculator, bill comes around $18K — $20K/year for a single server. Bringing in distributed system and fault tolerance would increase this cost quite significantly.
A Recap of Observability Costs
If you’ve made it this far, thank you for your attention. I trust that this exploration has shed light on your inquiries regarding the costs associated with observability. Make sure to check out our product in detail here